Frame vs. Trajectory Analyses of Pedestrian Dynamics Asymmetries in a Staircase Landing
DOI:
https://doi.org/10.17815/CD.2017.10Keywords:
pedestrian dynamics, high statistics measurements, statistical mechanics, data analysisAbstract
Real-life, out-of-laboratory, measurements of pedestrian walking dynamics allow extensive and fully-resolved statistical analyses. However, data acquisition in real-life is subjected to the randomness and heterogeneity that characterizes crowd flows over time. In a typical real-life location, disparate flow conditions follow one another in random order: for instance, a low density pedestrian co-flow dynamics may suddenly turn into a high density counter-flow scenario and then back again. Isolating occurrences of similar flow conditions within the acquired data is a paramount first step in the analyses in order to avoid spurious statistics and to enable qualitative comparisons.
In this paper we extend our previous investigation on the asymmetric pedestrian dynamics on a staircase landing, where we collected a large statistical database of measurements from ad hoc continuous recordings. This contribution has a two-fold aim: first, method-wise, we discuss an analysis workflow to consider large-scale experimental measurements, suggesting two querying approaches to automatically extract occurrences of similar flow scenarios out of datasets. These pursue aggregation of similar scenarios on either a frame or a trajectory basis. Second, we employ these two different perspectives to further explore asymmetries in the pedestrian dynamics in our measurement site. We report cross-comparisons of statistics of pedestrian positions, velocities and accelerations vs. flow conditions as well as vs. querying approach.
References
Corbetta, A., Lee, C., Muntean, A., Toschi, F.: Asymmetric pedestrian dynamics on a staircase landing from continuous measurements. In: Daamen, W., Knoop, V. (eds.) Traffic and Granular Flows '15, chap. 7. Springer (2016). doi:10.1007/978-3-319-33482-0_7
Karamouzas, I., Skinner, B., Guy, S.J.: Universal power law governing pedestrian interactions. Phys. Rev. Lett. 113, 238701 (2014). doi:10.1103/PhysRevLett.113.238701
Johansson, A., Helbing, D.: Analysis of empirical trajectory data of pedestrians. In: Pedestrian and Evacuation Dynamics 2008, pp. 203-214. Springer (2010). doi:10.1007/978-3-642-04504-2_15
Helbing, D., Mukerji, P.: Crowd disasters as systemic failures: analysis of the love parade disaster. EPJ Data Science 1(1), 1-40 (2012). doi:10.1140/epjds7
Johansson, A., Helbing, D., Shukla, P.: Specification of the social force pedestrian model by evolutionary adjustment to video tracking data. Adv. Complex Syst. 10(supp02), 271-288 (2007). doi:10.1142/S0219525907001355
Helbing, D., Johansson, A., Al-Abideen, H.Z.: Dynamics of crowd disasters: An empirical study. Phys. Rev. E 75(4), 046109 (2007). doi:10.1103/PhysRevE.75.046109
Zhang, J., Seyfried, A.: Comparison of intersecting pedestrian flows based on experiments. Physica A 405, 316-325 (2014). doi:10.1016/j.physa.2014.03.004
Brščić, D., Zanlungo, F., Kanda, T.: Density and velocity patterns during one year of pedestrian tracking. Transportation Research Procedia 2, 77-86 (2014). doi:10.1016/j.trpro.2014.09.011
Mehner, W., Boltes, M., Mathias, M., Leibe, B.: Robust marker-based tracking for measuring crowd dynamics. In: Computer Vision Systems, pp. 445-455. Springer (2015). doi:10.1007/978-3-319-20904-3_40
Boltes, M., Seyfried, A.: Collecting pedestrian trajectories. Neurocomputing 100, 127-133 (2013). doi:10.1016/j.neucom.2012.01.036
Zhang, J., Klingsch, W., Schadschneider, A., Seyfried, A.: Transitions in pedestrian fundamental diagrams of straight corridors and T-junctions. J. Stat. Mech.-Theory E. 2011(06), P06004 (2011). doi:10.1088/1742-5468/2011/06/P06004
Corbetta, A., Bruno, L., Muntean, A., Toschi, F.: High statistics measurements of pedestrian dynamics. Transportation Research Procedia 2, 96-104 (2014). doi:10.1016/j.trpro.2014.09.013
Seer, S., Brändle, N., Ratti, C.: Kinects and human kinetics: A new approach for studying pedestrian behavior. Transport. Res. C-Emer. 48, 212-228 (2014). doi:10.1016/j.trc.2014.08.012
Schadschneider, A., Klingsch, W., Klüpfel, H., Kretz, T., Rogsch, C., Seyfried, A.: Evacuation dynamics: Empirical results, modeling and applications. In: Encyclopedia of Complexity and Systems Science, pp. 3142-3176. Springer (2009). doi:10.1007/978-0-387-30440-3_187
Roggen, D., Wirz, M., Tröster, G., Helbing, D.: Recognition of crowd behavior from mobile sensors with pattern analysis and graph clustering methods. Netw. Heterog. Media 6(3), 521-544 (2011). doi:10.3934/nhm.2011.6.521
Corbetta, A.: Multiscale crowd dynamics: physical analysis, modeling and applications. Ph.D. thesis, Technische Universiteit Eindhoven (2016)
Corbetta, A., Lee, C., Benzi, R., Muntean, A., Toschi, F.: Fluctuations around mean walking behaviours in diluted pedestrian flows. Phys. Rev. E, in press, arXiv preprint arXiv:1610.07429 [physics.soc-ph] (2017)
Dalal, N., Triggs, B.: Histograms of oriented gradients for human detection. In: Computer Vision and Pattern Recognition, 2005. CVPR 2005. IEEE Computer Society Conference on, vol. 1, pp. 886-893. IEEE (2005). doi:10.1109/CVPR.2005.177
Cao, S., Zhang, J., Salden, D., Ma, J., Shi, C., Zhang, R.: Pedestrian dynamics in single-file movement of crowd with different age compositions. Phys. Rev. E 94, 012312 (2016). doi:10.1103/PhysRevE.94.012312
Garcimartín, A., Parisi, D., Pastor, J., Martín-Gómez, C., Zuriguel, I.: Flow of pedestrians through narrow doors with different competitiveness. J. Stat. Mech.-Theory E. 2016(4), 043402 (2016). doi:10.1088/1742-5468/2016/04/043402
Corbetta, A., Meeusen, J., Lee, C., Toschi, F.: Continuous measurements of real-life bidirectional pedestrian flows on a wide walkway. In: Pedestrian and Evacuation Dynamics 2016, pp. 18-24. University of Science and Technology of China press (2016)
Zanlungo, F., Brščić, D., Kanda, T.: Pedestrian group behaviour analysis under different density conditions. Transportation Research Procedia 2, 149-158 (2014). doi:10.1016/j.trpro.2014.09.020
Zanlungo, F., Ikeda, T., Kanda, T.: Potential for the dynamics of pedestrians in a socially interacting group. Phys. Rev. E 89, 012811 (2014). doi:10.1103/PhysRevE.89.012811
Tamura, Y., Terada, Y., Yamashita, A., Asama, H.: Modelling behaviour patterns of pedestrians for mobile robot trajectory generation. Int. J. Adv. Robot. Syst. 10 (2013). doi:10.5772/56668
Seitz, M., Seer, S., Klettner, S., Handel, O., Köster, G.: How do we wait? fundamentals, characteristics, and modeling implications. In: Daamen, W., Knoop, V. (eds.) Traffic and Granular Flows '15. Springer (2016)
Hoskins, B., Milke, J.: Study of Movement Speeds Down Stairs. SpringerBriefs in Fire. Springer New York (2012). URL https://books.google.nl/books?id=Jb27oMMfCiwC
Hoskins, B., Milke, J.: Differences in measurement methods for travel distance and area for estimates of occupant speed on stairs. Fire Safety J. 48, 49-57 (2012). doi:10.1016/j.firesaf.2011.12.009
Ronchi, E., Reneke, P., Kuligowski, E., Peacock, R.: An analysis of evacuation travel paths on stair landings by means of conditional probabilities. Fire Safety J. 65, 30-40 (2014). doi:10.1016/j.firesaf.2014.02.001
Peacock, R., Reneke, P., Kuligowski, E., Hagwood, C.: Movement on stairs during building evacuations. Fire Technol. pp. 1-27. doi:10.1007/s10694-016-0603-5
Moussaïd, M., Helbing, D., Garnier, S., Johansson, A., Combe, M., Theraulaz, G.: Experimental study of the behavioural mechanisms underlying self-organization in human crowds. Proc. R. Soc. B (276), 2755-2762 (2009). doi:10.1098/rspb.2009.0405
Fujiyama, T., Tyler, N.: Predicting the walking speed of pedestrians on stairs. Transport. Plan. Tech. 33(2), 177-202 (2010)
Bondy, J., Murty, U.: Graph theory with applications, vol. 290. Macmillan London (1976)
Hagberg, A., Schult, D., Swart, P.: Exploring network structure, dynamics, and function using NetworkX. In: Proceedings of the 7th Python in Science Conference (SciPy2008), pp. 11-15. Pasadena, CA USA (2008)
Helbing, D., Molnár, P.: Social force model for pedestrian dynamics. Phys. Rev. E 51(5), 4282-4286 (1995). doi:10.1103/PhysRevE.51.4282
Stanley, R.: Enumerative Combinatorics. Volume 2. Cambridge University Press (2001)
Corbetta, A., Muntean, A., Vafayi, K.: Parameter estimation of social forces in pedestrian dynamics models via a probabilistic method. Math. Biosci. Eng. 12(2), 337-356 (2015). doi:10.3934/mbe.2015.12.337
Microsoft Corp.: Kinect for Xbox 360 (2011). URL http://www.xbox.com/en-us/kinect/. Redmond, WA, USA.
The OpenPTV Consortium: OpenPTV: Open source particle tracking velocimetry (since 2012). URL http://www.openptv.net/
Willneff, J.: A spatio-temporal matching algorithm for 3d particle tracking velocimetry. Ph.D. thesis, ETH Zürich (2003)
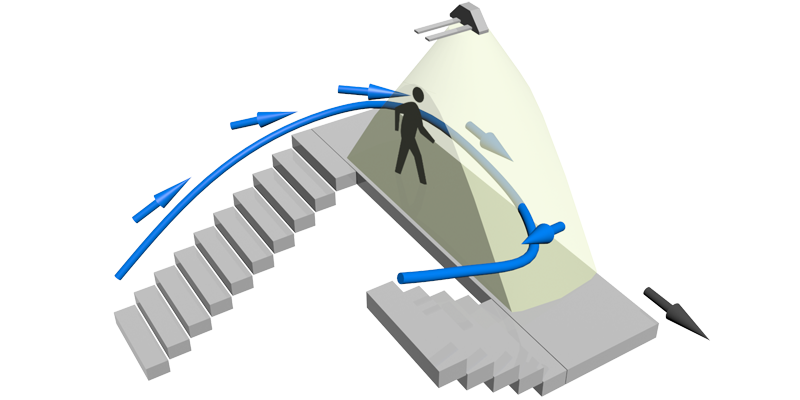
Downloads
Published
How to Cite
Issue
Section
License
Copyright (c) 2017 Alessandro Corbetta

This work is licensed under a Creative Commons Attribution 4.0 International License.
Authors contributing to Collective Dynamics agree to publish their articles under the Creative Commons Attribution 4.0 license.
This license allows:
Share — copy and redistribute the material in any medium or format
Adapt — remix, transform, and build upon the material
for any purpose, even commercially.
The licensor cannot revoke these freedoms as long as you follow the license terms.
Authors retain copyright of their work. They are permitted and encouraged to post items submitted to Collective Dynamics on personal or institutional websites and repositories, prior to and after publication (while providing the bibliographic details of that publication).