Towards a Reference Database for Pedestrian Destination Choice Model Development
DOI:
https://doi.org/10.17815/CD.2022.140Keywords:
Pedestrian destination choice, Reference database, Model calibration, Pedestrian dynamics, Choice modelling, Open dataAbstract
The move towards publishing research data openly has led to the formation of reference databases in many fields. The benefits of such resources are numerous, particularly in the development of models. While these exist in research on other aspects of pedestrian behaviour, no reference database is available for modelling pedestrian destination choice, the process by which pedestrians choose where they wish to visit next. This work seeks to construct such a database from the literature. The resulting data obtained are described and potential ways in which they could be used to calibrate a simple pedestrian destination choice model are presented. It contains four datasets that include destination choices for hundreds of pedestrians in settings ranging from university campuses and music festivals to highly structured stated preference surveys. A case study using one of these datasets to calibrate a simple pedestrian destination choice model is provided. These efforts highlight some general issues from creating and using reference data openly. Discussing these issues will hopefully guide the development of reference data and accelerate the development of accurate pedestrian destination choice models that can be applied generally.References
Miller J., H.: Activity-Based Analysis. In: Fischer, M.M., Nijkamp, P. (eds.) Hand book of Regional Science, vol. 2, pp. 705–724. Springer Berlin Heidelberg, Berlin (2014). doi:10.1007/978-3-662-60723-7_106
Institute of Advanced Simulation: Pedestrian Dynamics Data Archive (2017). URL https://ped.fz-juelich.de/da, accessed: 15/07/2022
Zhou, B., Tang, X., Wang, X.: Measuring Crowd Collectiveness. In: Proceedings of the 2013 IEEE Conference on Computer Vision and Pattern Recognition, CVPR ’13, pp. 3049–3056. IEEE Computer Society, USA (2013). doi:10.1109/CVPR.2013.392
Zhou, B.: Collective Motion Database (2013). URL http://mmlab.ie.cuhk.edu.hk/projects/collectiveness/dataset.htm, accessed: 15/07/2022
Wang, L., Li, G., Lei, J., Wang, T., Zhang, Y.: Density-Based Manifold Collective Clustering for Coherent Motion Detection. In: Proceedings of the International Conference on Machine Vision and Applications, ICMVA 2018, pp.22–27. Association for Computing Machinery, New York, NY, USA (2018). doi:10.1145/3220511.3220521
Gao, M.L., Wang, Y.T., Jiang, J., Shen, J., Zou, G.F., Liu, L.N.: Crowd motion segmentation via streak flow and collectiveness. In: 2017 Chinese Automation Congress (CAC), pp. 4067–4070 (2017). doi:10.1109/CAC.2017.8243492
Liu, W., Chan, A.B., Lau, R.W.H., Manocha, D.: Leveraging Long-Term Predictions and Online Learning in Agent-Based Multiple Person Tracking. IEEE Transactions on Circuits and Systems for Video Technology 25(3), 399–410 (2015). doi:10.1109/TCSVT.2014.2344511. Conference Name: IEEE Transactions on Circuits and Systems for Video Technology
Lin, W., Mi, Y., Wang, W., Wu, J., Wang, J., Mei, T.: A Diffusion and Clustering-Based Approach for Finding Coherent Motions and Understanding Crowd Scenes. IEEE Transactions on Image Processing 25(4), 1674–1687 (2016). doi:10.1109/TIP.2016.2531281. Conference Name: IEEE Transactions on Image Processing
Wu, S., Yang, H., Zheng, S., Su, H., Fan, Y., Yang, M.H.: Crowd Behavior Analysis via Curl and Divergence of Motion Trajectories. International Journal of Computer Vision 123(3), 499–519 (2017). doi:10.1007/s11263-017-1005-y
Mueller, J.P., Massaron, L.: Machine Learning for Dummies. John Wiley & Sons, Incorporated, Hoboken, United States (2016). URL http://ebookcentral.proquest.com/lib/bristol/detail.action?docID=4526803
Kotz, D., McDonald, C., Henderson, T., Gaughan, S., Proctor, P., Shubina, A., Yeo, J., Akestoridis, D.G., Cannon, H., Dimov, R., Jacobson, J., Johnson, S., Kelk, K., Malajian, D., Muckle-Jones, R., Neophytou, C., Pietikainen, P., Riina, A., Seletsky, O.: CRAWDAD. URL https://crawdad.org/about.html, accessed: 31/01/2022
Sarafraz, A.: Datasets (2020). URL https://computervisiononline.com/datasets, accessed: 31/01/2022
University College San Diego: Metabolomics Workbench. URL https://www.metabolomicsworkbench.org/, accessed: 14/07/2022
Seyfried, A., Passon, O., Steffen, B., Boltes, M., Rupprecht, T., Klingsch, W.: New Insights into Pedestrian Flow Through Bottlenecks. Transportation Science 43(3), 395–406 (2009). doi:10.1287/trsc.1090.0263. Publisher: INFORMS
Godel, M., Bode, N.W.F., K ¨ oster, G., Bungatz, H.J.: Bayesian inference methods to calibrate crowd dynamics models for safety applications. Safety Science 147, 105586 (2022). doi:10.1016/j.ssci.2021.105586. Publisher: Elsevier
Cao, R.F., Lee, E.W.M., Yuen, A.C.Y., Chen, T.B.Y., De Cachinho Cordeiro, I.M., Shi, M., Wei, X., Yeoh, G.H.: Simulation of competitive and cooperative egress movements on the crowd emergency evacuation. Simulation Modelling Practice and Theory 109, 102309 (2021). doi:10.1016/j.simpat.2021.102309
Haghpanah, F., Ghobadi, K., Schafer, B.W.: Multi-hazard hospital evacuation planning during disease outbreaks using agent-based modeling. International Journal of Disaster Risk Reduction 66, 102632 (2021). doi:10.1016/j.ijdrr.2021.102632
Li, N., Guo, R.Y.: Simulation of bi-directional pedestrian flow through a bottleneck: Cell transmission model. Physica A: Statistical Mechanics and its Applications 555, 124542 (2020). doi:10.1016/j.physa.2020.124542
Zhuang, Y., Liu, Z., Schadschneider, A., Yang, L., Huang, J.: Exploring the behavior of self-organized queuing for pedestrian flow through a non-service bottleneck. Physica A: Statistical Mechanics and its Applications 562, 125186 (2021). doi:10.1016/j.physa.2020.125186
Li, H., Zhang, J., Yang, L., Song, W., Yuen, K.K.R.: A comparative study on the bottleneck flow between preschool children and adults under different movement motivations. Safety Science 121, 30–41 (2020). doi:10.1016/j.ssci.2019.09.002
Cao, S., Seyfried, A., Zhang, J., Holl, S., Song, W.: Fundamental diagrams for multidirectional pedestrian flows. Journal of Statistical Mechanics: Theory and Experiment 2017(3), 033404 (2017). doi:10.1088/1742-5468/aa620d. Publisher: IOP Publishing
Xu, Q., Chraibi, M., Seyfried, A.: Anticipation in a velocity-based model for pedestrian dynamics. Transportation Research Part C: Emerging Technologies 133, 103464 (2021). doi:10.1016/j.trc.2021.103464
Zhang, J., Klingsch, W., Schadschneider, A., Seyfried, A.: Transitions in pedestrian fundamental diagrams of straight corridors and T-junctions. Journal of Statistical Mechanics: Theory and Experiment 2011(06), P06004 (2011). doi:10.1088/1742-5468/2011/06/P06004. Publisher: IOP Publishing
Rathinakumar, K., Quaini, A.: A microscopic approach to study the onset of a highly infectious disease spreading. Mathematical Biosciences 329, 108475 (2020). doi:10.1016/j.mbs.2020.108475
Zhao, X., Zhang, J., Song, W.: A Radar-Nearest-Neighbor based data-driven approach for crowd simulation. Transportation Research Part C: Emerging Technologies 129, 103260 (2021). doi:10.1016/j.trc.2021.103260
Munafo, M.R., Nosek, B.A., Bishop, D.V.M., Button, K.S., Chambers, C.D., Percie du Sert, N., Simonsohn, U., Wagenmakers, E.J., Ware, J.J., Ioannidis, J.P.A.: A manifesto for reproducible science. Nature Human Behaviour 1(1), 1–9 (2017). doi:10.1038/s41562-016-0021. Number: 1 Publisher: Nature Publishing Group
Wilkinson, M.D., Dumontier, M., Aalbersberg, I.J., Appleton, G., Axton, M., Baak, A., Blomberg, N., Boiten, J.W., da Silva Santos, L.B., Bourne, P.E., Bouwman, J., Brookes, A.J., Clark, T., Crosas, M., Dillo, I., Dumon, O., Edmunds, S., Evelo, C.T., Finkers, R., Gonzalez-Beltran, A., Gray, A.J.G., Groth, P., Goble, C., Grethe, J.S., Heringa, J., ’t Hoen, P.A.C., Hooft, R., Kuhn, T., Kok, R., Kok, J., Lusher, S.J., Martone, M.E., Mons, A., Packer, A.L., Persson, B., Rocca-Serra, P., Roos, M., van Schaik, R., Sansone, S.A., Schultes, E., Sengstag, T., Slater, T., Strawn, G., Swertz, M.A., Thompson, M., van der Lei, J., van Mulligen, E., Velterop, J., Waagmeester, A., Wittenburg, P., Wolstencroft, K., Zhao, J., Mons, B.: The FAIR Guiding Principles for scientific data management and stewardship. Scientific Data 3(1), 160018 (2016). doi:10.1038/sdata.2016.18. Number: 1 Publisher: Nature Publishing Group
Moher, D., Liberati, A., Tetzlaff, J., Altman, D.G., Group, T.P.: Preferred Reporting Items for Systematic Reviews and Meta-Analyses: The PRISMA Statement. PLOS Medicine 6(7), e1000097 (2009). doi:10.1371/journal.pmed.1000097. Publisher: Public Library of Science
Wee, B.V., Banister, D.: How to Write a Literature Review Paper? Transport Reviews 36(2), 278–288 (2016). doi:10.1080/01441647.2015.1065456. Publisher: Routledge
Kitchenam, B., Charters, S.: Guidelines for performing Systematic Literature Reviews in software engineering. Technical, University of Durham, Durham, UK (2007). URL https://www.researchgate.net/publication/258968007_Kitchenham_B_Guidelines_for_performing_ Systematic_Literature_Reviews_in_software_engineering_ EBSE_Technical_Report_EBSE-2007-01
Greenhalgh, T., Peacock, R.: Effectiveness and efficiency of search methods in systematic reviews of complex evidence: audit of primary sources. BMJ (Clinical research ed.) 331(7524), 1064–1065 (2005). doi:10.1136/bmj.38636.593461.68
Feng, Y., Duives, D.C., Daamen, W., Hoogendoorn, S.P.: Data collection methods for studying pedestrian behaviour: A systematic review. Building and Environment 187, 107329 (2021). doi:10.1016/j.buildenv.2020.107329
Seaborn, K., Miyake, N.P., Pennefather, P., Otake-Matsuura, M.: Voice in Human–Agent Interaction. ACM Computing Surveys 54(4), 1–43 (2022). doi:10.1145/3386867
Xu, M., Nie, X., Li, H., Cheng, J.C., Mei, Z.: Smart construction sites: A promising approach to improving on-site HSE management performance. Journal of Building Engineering 49, 104007 (2022). doi:10.1016/j.jobe.2022.104007
Jalali, S., Wohlin, C.: Systematic literature studies: Database searches vs. backward snowballing. In: Proceedings of the 2012 ACM-IEEE International Symposium on Empirical Software Engineering and Measurement, pp. 29–38. IEEE, Lund, Sweden (2012). doi:10.1145/2372251.2372257
Ying, F., Wallis, A.O.G., Beguerisse-D´ıaz, M., Porter, M.A., Howison, S.D.: Customer mobility and congestion in supermarkets. Physical Review E 100 (2019). doi:10.1103/PhysRevE.100.062304. ArXiv: 1905.13098
Arentze, T.A., Timmermans, H.J.P.: Deriving performance indicators from models of multipurpose shopping behavior. Journal of Retailing and Consumer Services 8(6), 325–334 (2001). doi:10.1016/S0969-6989(00)00038-2
Beaulieu, A., Farooq, B.: A dynamic mixed logit model with agent effect for pedestrian next location choice using ubiquitous Wi-Fi network data. International Journal of Transportation Science and Technology 8(3), 280–289 (2019). doi:10.1016/j.ijtst.2019.02.003
Ettema, D., Bastin, F., Polak, J., Ashiru, O.: Modelling the joint choice of activity timing and duration. Transportation Research Part A: Policy and Practice 41(9), 827–841 (2007). doi:10.1016/j.tra.2007.03.001
Hui, S.K., Bradlow, E.T., Fader, P.S.: Testing Behavioral Hypotheses Using an Integrated Model of Grocery Store Shopping Path and Purchase Behavior. Journal of Consumer Research 36(3), 478–493 (2009). doi:10.1086/599046
Danalet, A.: Activity choice modeling for pedestrian facilities. Ph.D. thesis, Swiss Federal Institute of Technology, Lausanne (2015). URL https://infoscience.epfl.ch/record/214544#
Tinguely, L.: Exploiting pedestrian WiFi traces for destination choice modeling. Master’s thesis, EPFL, Lausanne, Switzerland (2015). URL https://infoscience.epfl.ch/record/209732
Vukadinovic, V., Dreier, F., Mangold, S.: A simple framework to simulate the mobility and activity of theme park visitors. In: Proceedings of the 2011 Winter Simulation Conference (WSC), pp. 3248–3260 (2011). doi:10.1109/WSC.2011.6148022
Yao, R., Bekhor, S.: Data-driven choice set generation and estimation of route choice models. Transportation Research Part C: Emerging Technologies 121, 102832 (2020). doi:10.1016/j.trc.2020.102832
Zhu, W., Timmermans, H.J.P.: Modeling pedestrian shopping behavior using principles of bounded rationality: model comparison and validation. Journal of Geographical Systems 13(2), 101–126 (2011). doi:10.1007/s10109-010-0122-8
Duives, D.C., Daamen, W., Hoogendoorn, S.P.: State-of-the-art crowd motion simulation models. Transportation Research Part C: Emerging Technologies 37, 193–209 (2013). doi:10.1016/j.trc.2013.02.005
Feliciani, C., Nishinari, K.: Estimation of pedestrian crowds’ properties using commercial tablets and smartphones. Transportmetrica B: Transport Dynamics 7(1), 865–896 (2019). doi:10.1080/21680566.2018.1517061. Publisher: Taylor & Francis
Voskamp, H.a.W.: Measuring the influence of congested bottleneck on route choice behavior of pedestrians at Utrecht Centraal. Master’s thesis, Delft University of Technology, Delft (2012). URL http://resolver.tudelft.nl/uuid:6a272595-d98b-4769-99b4-1eec7ab2b620
Bigano, A., Hamilton, J.M., Tol, R.S.J.: The Impact of Climate on Holiday Destination Choice. Climatic Change 76(3), 389–406 (2006). doi:10.1007/s10584-005-9015-0
Karl, M., Reintinger, C., Schmude, J.: Reject or select: Mapping destination choice. Annals of Tourism Research 54, 48–64 (2015). doi:10.1016/j.annals.2015.06.003
Fotheringham, A.S.: Modelling Hierarchical Destination Choice. Environment and Planning A: Economy and Space 18(3), 401–418 (1986). doi:10.1068/a180401
Andion, J., Navarro, J.M., L ´ opez, G., ´ Alvarez Campana, M., Due ´ 34 nas, J.C.: Smart Behavioral Analytics over a Low-Cost IoT Wi-Fi Tracking Real Deployment. Wireless Communications and Mobile Computing 2018, e3136471 (2018). doi:10.1155/2018/3136471. Publisher: Hindawi
Raun, J., Ahas, R., Tiru, M.: Measuring tourism destinations using mobile tracking data. Tourism Management 57, 202–212 (2016). doi:10.1016/j.tourman.2016.06.006
Andresen, E., Chraibi, M., Seyfried, A.: A representation of partial spatial knowledge: a cognitive map approach for evacuation simulations. Transportmetrica A: Transport Science 14(5-6), 433–467 (2018). doi:10.1080/23249935.2018.1432717
Tong, Y., Bode, N.W.F.: Higher investment levels into pre-planned routes increase the adherence of pedestrians to them. Transportation Research Part F: Traffic Psychology and Behaviour 82, 297–315 (2021). doi:10.1016/j.trf.2021.07.019
Shao, W., Terzopoulos, D.: Autonomous Pedestrians. In: Proceedings of the 2005 ACM SIGGRAPH/Eurographics Symposium on Computer Animation, SCA ’05, pp. 19–28. ACM, New York, NY, USA (2005). doi:10.1145/1073368.1073371
Axhausen, K.W., Zimmermann, A., Schonfelder, S., Rindsf ¨ user, G., Haupt, T.: Observing the rhythms of daily life: A six-week travel diary. Transportation 29(2), 95–124 (2002). doi:10.1023/A:1014247822322
Shiftan, Y.: Practical Approach to Model Trip Chaining. Transportation Research Record 1645(1), 17–23 (1998). doi:10.3141/1645-03
Danalet, A., Farooq, B., Bierlaire, M.: A Bayesian approach to detect pedestrian destination-sequences from WiFi signatures. Transportation Research Part C: Emerging Technologies 44, 146–170 (2014). doi:10.1016/j.trc.2014.03.015
Danalet, A., Bierlaire, M.: A path choice approach to activity modeling with a pedestrian case study. p. 45. Unpublished, Monte Verita, Ascona, Switzerland (2014). doi:10.13140/2.1.4099.8728
Danalet, A., Tinguely, L., Lapparent, M.d., Bierlaire, M.: Location choice with longitudinal WiFi data. Journal of Choice Modelling 18, 1–17 (2016). doi:10.1016/j.jocm.2016.04.003
Bonne, B., Barzan, A., Quax, P., Lamotte, W.: WiFiPi: Involuntary tracking of visitors at mass events. In: 2013 IEEE 14th International Symposium on "A World of Wireless, Mobile and Multimedia Networks” (WoWMoM), pp. 1–6. IEEE, Madrid, Spain (2013). doi:10.1109/WoWMoM.2013.6583443
King, C., Bode, N.W.F.: A virtual experiment on pedestrian destination choice: the role of schedules, the environment and behavioural categories. Royal Society Open Science 9(7), 211982 (2022). doi:10.1098/rsos.211982
Train, K.: Discrete choice methods with simulation, 2nd ed edn. Cambridge University Press, Cambridge; New York (2009). URL https://eml.berkeley.edu/books/train1201.pdf. OCLC: ocn349248337
Hoogendoorn, S.P., Bovy, P.H.L.: Pedestrian route-choice and activity scheduling theory and models. Transportation Research Part B: Methodological 38(2), 169–190 (2004). doi:10.1016/S0191-2615(03)00007-9
King, C., Koltsova, O., Bode, N.W.F.: Simulating the effect of measurement errors on pedestrian destination choice model calibration. Transportmetrica A: Transport Science pp. 1–41 (2022). doi:10.1080/23249935.2021.2017510. Publisher: Taylor & Francis
Kielar, P.M., Borrmann, A.: Modeling pedestrians’ interest in locations: A concept to improve simulations of pedestrian destination choice. Simulation Modelling Practice and Theory 61, 47–62 (2016). doi:10.1016/j.simpat.2015.11.003
Saarloos, D., Fujiwara, A., Zhang, J.: The Interaction between Pedestrians and Facilities in Central Business Districts: An Explorative Case Study. Journal of the Eastern Asia Society for Transportation Studies 7(0), 1870–1885 (2007). doi:10.11175/easts.7.1870. Publisher: Eastern Asia Society for Transportation Studies
Kwak, J., Jo, H.H., Luttinen, T., Kosonen, I.: Modeling Pedestrian Switching Behavior for Attractions. Transportation Research Procedia 2, 612–617 (2014). doi:10.1016/j.trpro.2014.09.102
Arentze, T.A., Ettema, D., Timmermans, H.J.P.: Location choice in the context of multi-day activity-travel patterns: model development and empirical results. Transportmetrica A: Transport Science 9(2), 107–123 (2013). doi:10.1080/18128602.2010.538870
Ettema, D., Borgers, A.W.J., Timmermans, H.J.P.: Simulation model of activity scheduling behavior. Transportation Research Record 1413, 1–11 (1993). URL https://www.persistent-identifier.nl/urn:nbn:nl:ui:25-0287ad73-721b-4094-962c-fa7cc87db416
Fesenmaier, D.R.: Integrating activity patterns into destination choice models. Journal of Leisure Research 20(3), 175–191 (1988)
Kurose, S., Borgers, A.W.J., Timmermans, H.J.P.: Classifying Pedestrian Shopping Behaviour According to Implied Heuristic Choice Rules. Environment and Planning B: Planning and Design 28(3), 405–418 (2001). doi:10.1068/b2622
Ton, D.: Navistation: A study into the route and activity location choice behaviour of departing pedestrians in train stations. Ph.D. thesis, Delft University of Technology (2014)
van der Hagen, X., Borgers, A.W.J., Timmermans, H.J.P.: Spatiotemporal Sequencing Processes of Pedestrian in Urban Retail Environments. The Journal of the RSAI 70(1), 37–52 (1991). doi:10.1007/BF01463442
Zhu, W., Timmermans, H.J.P., De, W.: Temporal Variation in Consumer Spatial Behavior in Shopping Streets. Journal of Urban Planning and Development 132(3), 166–171 (2006). doi:10.1061/(ASCE)0733-9488(2006)132:3(166)
Fahmy, S.A., Alablani, B.A., Abdelmaguid, T.F.: Shopping center design using a facility layout assignment approach. In: 2014 9th International Conference on Informatics and Systems, pp. ORDS–1–ORDS–7. IEEE, Cairo, Egypt (2014). doi:10.1109/INFOS.2014.7036689
Yang, Y., Fik, T., Zhang, J.: Modeling Sequential Tourist Flows:Where is the Next Destination? Annals of Tourism Research 43, 297–320 (2013). doi:10.1016/j.annals.2013.07.005
Dijkstra, J., Timmermans, H.J.P., de Vries, B.: Activation of Shopping Pedestrian Agents—Empirical Estimation Results. Applied Spatial Analysis and Policy 6(4), 255–266 (2013). doi:10.1007/s12061-012-9082-3
Greenwood, D., Sharma, S., Johansson, A.: Mobility Modelling in a Process Constrained Environment: Modelling the Movements of Nurses in a Neonatal Intensive Care Unit. In: Chraibi, M., Boltes, M., Schadschneider, A., Seyfried, A. (eds.) Traffic and Granular Flow ’13, pp. 233–241. Springer International Publishing, Cham (2015). URL https://www.semanticscholar.org/paper/Mobility-Modelling-in-a-Process-Constrained-the-of-Greenwood-Sharma/f8a8c139eb0657f6f2863df4419ed6cd43b8a11
R Core Team: R: A language and environment for statistical computing (2020). URL https://www.R-project.org/
Cisco Meraki: Understanding Wireless Performance and Coverage. Technical (2020). URL https://documentation.meraki.com/MR/WiFi_Basics_and_Best_Practices/Understanding_Wireless_Performance_and_Coverage
Chilipirea, C., Dobre, C., Baratchi, M., Steen, M.v.: Identifying Movements in Noisy Crowd Analytics Data. In: 2018 19th IEEE International Conference on Mobile Data Management (MDM), pp. 161–166 (2018). doi:10.1109/MDM.2018.00033. ISSN: 2375-0324
Kern, W.: Solid Mensuration With Proofs (1938). URL http://archive.org/details/in.ernet.dli.2015.205959
Vincenty, T.: Direct and Inverse Solutions of Geodesics on the Ellipsoid with Application of Nested Equations. Survey Review 23(176), 88–93 (1975). doi:10.1179/sre.1975.23.176.88. Publisher: Taylor & Franci
National Geospatial-intelligence Agency: NGA Geomatics - WGS 84. URL https://earth-info.nga.mil/index.php?dir=wgs84&action=wgs84
Zhai, Y., Baran, P.K., Wu, C.: Spatial distributions and use patterns of user groups in urban forest parks: An examination utilizing GPS tracker. Urban Forestry & Urban Greening 35, 32–44 (2018). doi:10.1016/j.ufug.2018.07.014
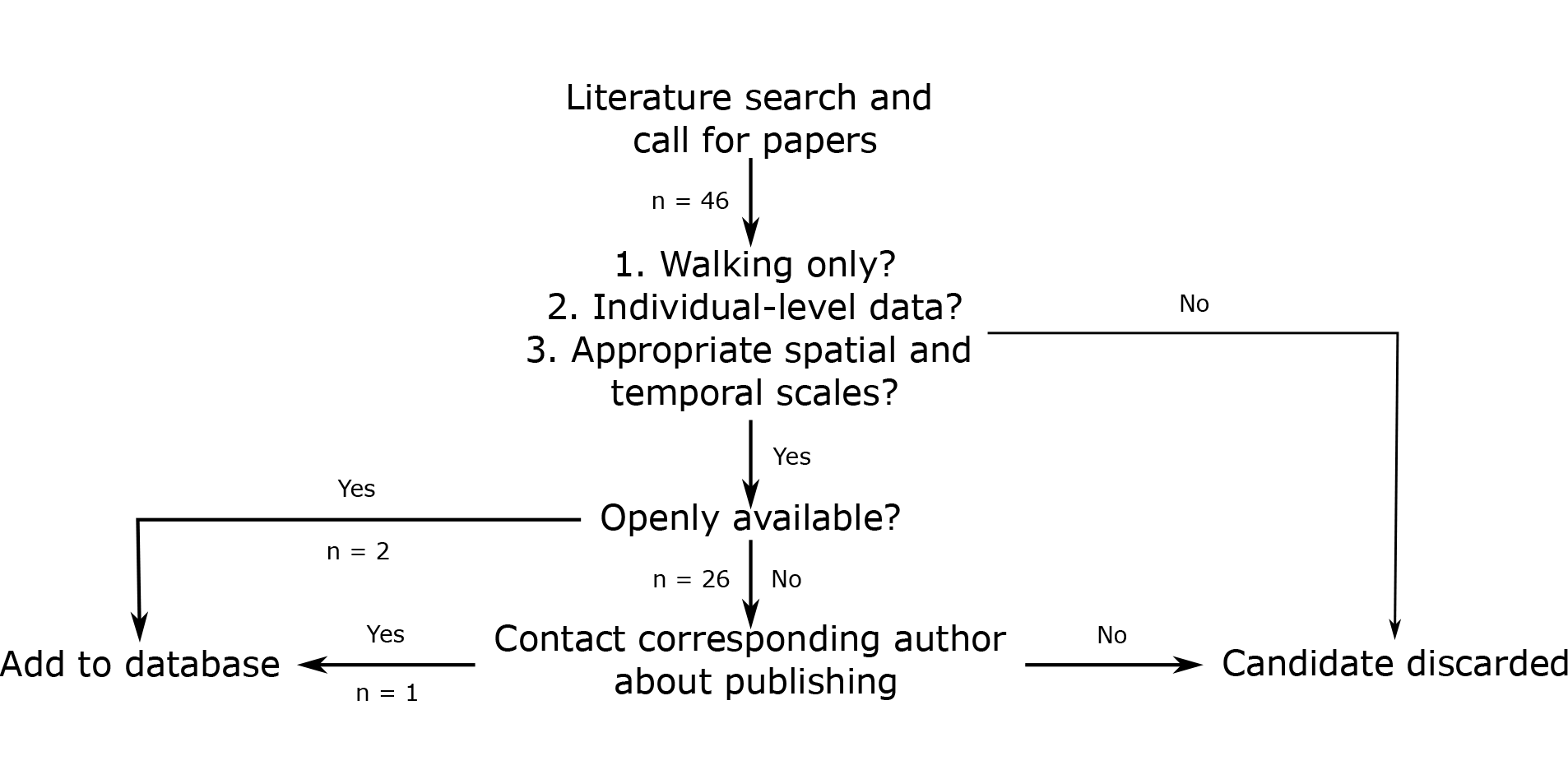
Downloads
Published
How to Cite
Issue
Section
License
Copyright (c) 2022 Christopher King, Nikolai Bode

This work is licensed under a Creative Commons Attribution 4.0 International License.
Authors contributing to Collective Dynamics agree to publish their articles under the Creative Commons Attribution 4.0 license.
This license allows:
Share — copy and redistribute the material in any medium or format
Adapt — remix, transform, and build upon the material
for any purpose, even commercially.
The licensor cannot revoke these freedoms as long as you follow the license terms.
Authors retain copyright of their work. They are permitted and encouraged to post items submitted to Collective Dynamics on personal or institutional websites and repositories, prior to and after publication (while providing the bibliographic details of that publication).