Video Analytics for Understanding Pedestrian Mobility Patterns in Public Spaces: The Case of Milan
DOI:
https://doi.org/10.17815/CD.2024.172Keywords:
Pedestrians, Public spaces, Videos analysis, Computer visionAbstract
The main objective of this research was to characterize public spaces through a mobility study on pedestrian patterns analyzed by means of video analytics (i.e., object detection, crowd counting, pedestrian tracking), for the case study of Piazza Duomo (Milan, Italy). The analysis focused on defining different pedestrian profiles through observable behavioural parameters (e.g., density conditions, speeds, trajectories, etc.). The results of the research could support the definition of an evidence-based approach for regeneration projects of urban public spaces.
References
Zhang, F., Miranda, A., Duarte, F., Vale, L., Hack, G., Liu, Y., Batty, M., Ratti, C.: Urban visual intelligence: Studying cities with ai and street-level imagery (2023). doi:10.48550/arXiv.2301.00580
Hou, J., Chenid, L., Zhang, E., Jia, H., Longid, Y.: Quantifying the usage of small public spaces using deep convolutional neural network. PLoS ONE 15 (2020). doi:10.1371/journal.pone.0239390
Messa, F., Ceccarelli, G., Gorrini, A., Presicce, D., Choubassi, Choubassi: Deep learning video analytics to assess vga measures and proxemic behaviour in public spaces. In: 13th International Space Syntax Symposium (13SSS), 22-24 June 2022, Bergen (Norway), pp. 1-22 (2022)
Lin, M., Hsu, W.J.: Mining gps data for mobility patterns: A survey. Pervasive and mobile computing 12, 1-16 (2014). doi:10.1016/j.pmcj.2013.06.005
Gorrini, A., Messa, F., Ceccarelli, G., Choubassi, R.: Covid-19 pandemic and activity patterns in milan. wi-fi sensors and location-based data. TeMA-Journal of Land Use, Mobility and Environment 14(2), 211-226 (2021). doi:10.6093/1970-9870/7886
Farrokhtala, A., Chen, Y., Hu, T., Ye, S.: Toward understanding hidden patterns in human mobility using wi-fi. In: 2018 IEEE Canadian Conference on Electrical & Computer Engineering (CCECE), pp. 1-4 (2018). doi:10.1109/CCECE.2018.8447727
Ceccarelli, G., Messa, F., Gorrini, A., Presicce, D., Choubassi, R.: Deep learning video analytics for the assessment of street experiments: The case of bologna. Journal of Urban Mobility (2023). Submitted
Niu, T., Qing, L., Han, L., Long, Y., Hou, J., Li, L., Tang, W., Teng, Q.: Small public space vitality analysis and evaluation based on human trajectory modeling using video data. Building and Environment 225, 109563 (2022). doi:10.1016/j.buildenv.2022.109563
Tripathi, G., Singh, K., Vishwakarma, D.K.: Convolutional neural networks for crowd behaviour analysis: a survey. The Visual Computer 35, 753-776 (2019). doi:10.1007/s00371-018-1499-5
Zou, Z., Chen, K., Shi, Z., Guo, Y., Ye, J.: Object detection in 20 years: A survey. Proceedings of the IEEE 111(3), 257-276 (2023). doi:10.1109/JPROC.2023.3238524
Aziz, L., Haji Salam, M.S.B., Sheikh, U.U., Ayub, S.: Exploring deep learning-based architecture, strategies, applications and current trends in generic object detection: A comprehensive review. IEEE Access 8, 170461-170495 (2020). doi:10.1109/ACCESS.2020.3021508
Liu, L., Ouyang, W., Wang, X., Fieguth, P., Chen, J., Liu, X., Pietikäinen, M.: Deep learning for generic object detection: A survey. International journal of computer vision 128, 261-318 (2020). doi:10.48550/arXiv.1809.02165
Ciaparrone, G., Sánchez, F.L., Tabik, S., Troiano, L., Tagliaferri, R., Herrera, F.: Deep learning in video multi-object tracking: A survey. Neurocomputing 381, 61-88 (2020). doi:10.1016/j.neucom.2019.11.023
Zhao, Z.Q., Zheng, P., Xu, S.T., Wu, X.: Object detection with deep learning: A review. IEEE Transactions on Neural Networks and Learning Systems PP, 1-21 (2019). doi:10.1109/TNNLS.2018.2876865
Jiao, L., Zhang, F., Liu, F., Yang, S., Li, L., Feng, Z., Qu, R.: A survey of deep learning-based object detection. IEEE access 7, 128837-128868 (2019). doi:10.1109/ACCESS.2019.2939201
Tomè, D., Monti, F., Baroffio, L., Bondi, L., Tagliasacchi, M., Tubaro, S.: Deep convolutional neural networks for pedestrian detection. Signal processing: image communication 47, 482-489 (2016). doi:10.1016/j.image.2016.05.007
Jiang, K., Xie, T., Yan, R., Wen, X., Li, D., Jiang, H., Jiang, N., Feng, L., Duan, X., Wang, J.: An attention mechanism-improved yolov7 object detection algorithm for hemp duck count estimation. Agriculture 12(10), 1659 (2022). doi:10.3390/agriculture12101659
Bewley, A., Ge, Z., Ott, L., Ramos, F., Upcroft, B.: Simple online and realtime tracking. In: 2016 IEEE international conference on image processing (ICIP), pp. 3464-3468. IEEE (2016). doi:10.1109/ICIP.2016.7533003
Fruin, J.: Pedestrian Planning and Design. Metropolitan Association of Urban Designers and Environmental Planners (1971). URL https://books.google.it/books?id=AydSAAAAMAAJ
Gorrini, A., Vizzari, G., Bandini, S.: Age and group-driven pedestrian behaviour: from observations to simulations. Collective Dynamics 1, 1–16 (2016). doi:10.17815/CD.2016.3
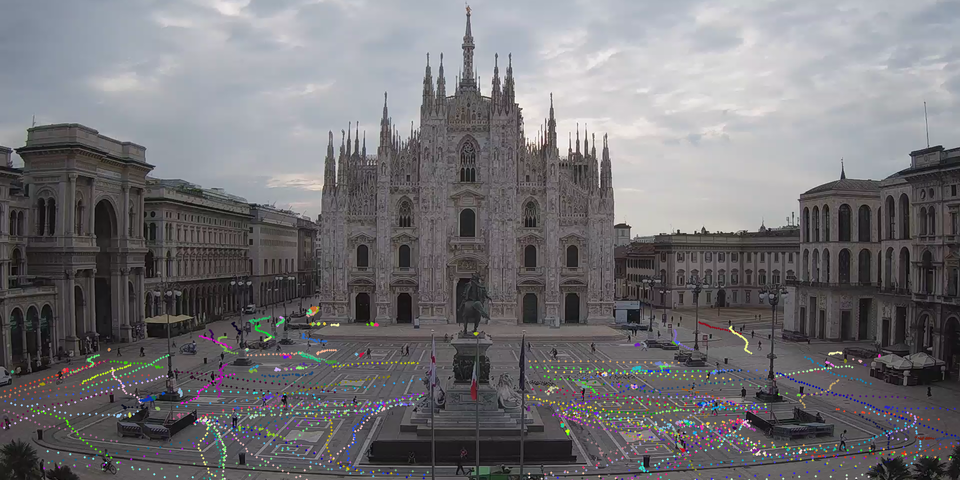
Downloads
Published
How to Cite
Issue
Section
License
Copyright (c) 2024 Lorenzo Lorgna, Giulia Ceccarelli , Andrea Gorrini, Michele Ciavotta

This work is licensed under a Creative Commons Attribution 4.0 International License.
Authors contributing to Collective Dynamics agree to publish their articles under the Creative Commons Attribution 4.0 license.
This license allows:
Share — copy and redistribute the material in any medium or format
Adapt — remix, transform, and build upon the material
for any purpose, even commercially.
The licensor cannot revoke these freedoms as long as you follow the license terms.
Authors retain copyright of their work. They are permitted and encouraged to post items submitted to Collective Dynamics on personal or institutional websites and repositories, prior to and after publication (while providing the bibliographic details of that publication).