Improving Pedestrian Dynamics Predictions Using Neighboring Factors
DOI:
https://doi.org/10.17815/CD.2024.178Keywords:
Pedestrian Dynamics, Speed Predictions, Neighboriing Effects, Neural NetworksAbstract
Predicting pedestrian dynamics is a complex task as pedestrian speed is influenced by various external factors. This study investigates neighboring factors that can be used to improve pedestrian walking speed prediction accuracy in both low- and high-density scenarios. Different factors are proposed, including Mean Distance, Time-to-Collision, and Front Effect, and data for each factor is extracted from different public datasets. The collected data at time t is used to train a neural network to predict the pedestrian walking speed at time t + ∆t. Predictions are evaluated using the Mean Absolute Error. Our results demonstrate that incorporating the Front Effect significantly improves prediction accuracy in both low- and high-density scenarios, whereas the Mean Distance factor only proves effective in high-density cases. On the other hand, no significant improvement is observed when considering the Time-to-Collision factor. These preliminary findings can be utilized to enhance the accuracy of pedestrian dynamics predictions by incorporating these factors as additional features within the model.
References
Nicolas, A., Hassan, F.H.: Social groups in pedestrian crowds: review of their influence on the dynamics and their modelling. Transportmetrica A: transport science 19(1), 1970651 (2023)
Seyfried, A., Steffen, B., Klingsch, W., Boltes, M.: The fundamental diagram of pedestrian movement revisited. Journal of Statistical Mechanics: Theory and Experiment 2005(10), P10002 (2005)
Franvek, M.: Environmental factors influencing pedestrian walking speed. Perceptual and motor skills 116(3), 992-1019 (2013)
Meeder, M., Aebi, T., Weidmann, U.: The influence of slope on walking activity and the pedestrian modal share. Transportation research procedia 27, 141-147 (2017)
Xue, H., Huynh, D.Q., Reynolds, M.: Ss-lstm: A hierarchical lstm model for pedestrian trajectory prediction. In: 2018 IEEE Winter Conference on Applications of Computer Vision (WACV), pp. 1186-1194. IEEE (2018)
Vemula, A., Muelling, K., Oh, J.: Social attention: Modeling attention in human crowds. In: 2018 IEEE international Conference on Robotics and Automation (ICRA), pp. 4601-4607. IEEE (2018)
Peng, Y., Zhang, G., Shi, J., Xu, B., Zheng, L.: Srai-lstm: A social relation attention-based interaction-aware lstm for human trajectory prediction. Neurocomputing 490, 258-268 (2022)
Dang, H.T., Korbmacher, R., Tordeux, A., Gaudou, B., Verstaevel, N.: Ttc-slstm: Human trajectory prediction using time-to-collision interaction energy. In: 2023 15th International Conference on Knowledge and Systems Engineering (KSE), pp. 1-6. IEEE (2023)
Korbmacher, R., Dang, H.T., Tordeux, A.: Predicting pedestrian trajectories at different densities: A multi-criteria empirical analysis. Physica A: Statistical Mechanics and its Applications 634, 129440 (2024)
Karamouzas, I., Skinner, B., Guy, S.J.: Universal power law governing pedestrian interactions. Physical review letters 113(23), 238701 (2014)
Duives, D.C., Daamen, W., Hoogendoorn, S.P.: State-of-the-art crowd motion simulation models. Transportation research part C: emerging technologies 37, 193-209 (2013)
Pellegrini, S., Ess, A., Schindler, K., Van Gool, L.: You'll never walk alone: Modeling social behavior for multi-target tracking. In: 2009 IEEE 12th international conference on computer vision, pp. 261-268. IEEE (2009)
Lerner, A., Chrysanthou, Y., Lischinski, D.: Crowds by example. In: Computer graphics forum, vol. 26, pp. 655-664. Wiley Online Library (2007)
Cao, S., Seyfried, A., Zhang, J., Holl, S., Song, W.: Fundamental diagrams for multidirectional pedestrian flows. Journal of Statistical Mechanics: Theory and Experiment 2017(3), 033404 (2017)
Tordeux, A., Chraibi, M., Seyfried, A., Schadschneider, A.: Prediction of pedestrian dynamics in complex architectures with artificial neural networks. Journal of intelligent transportation systems 24(6), 556-568 (2020)
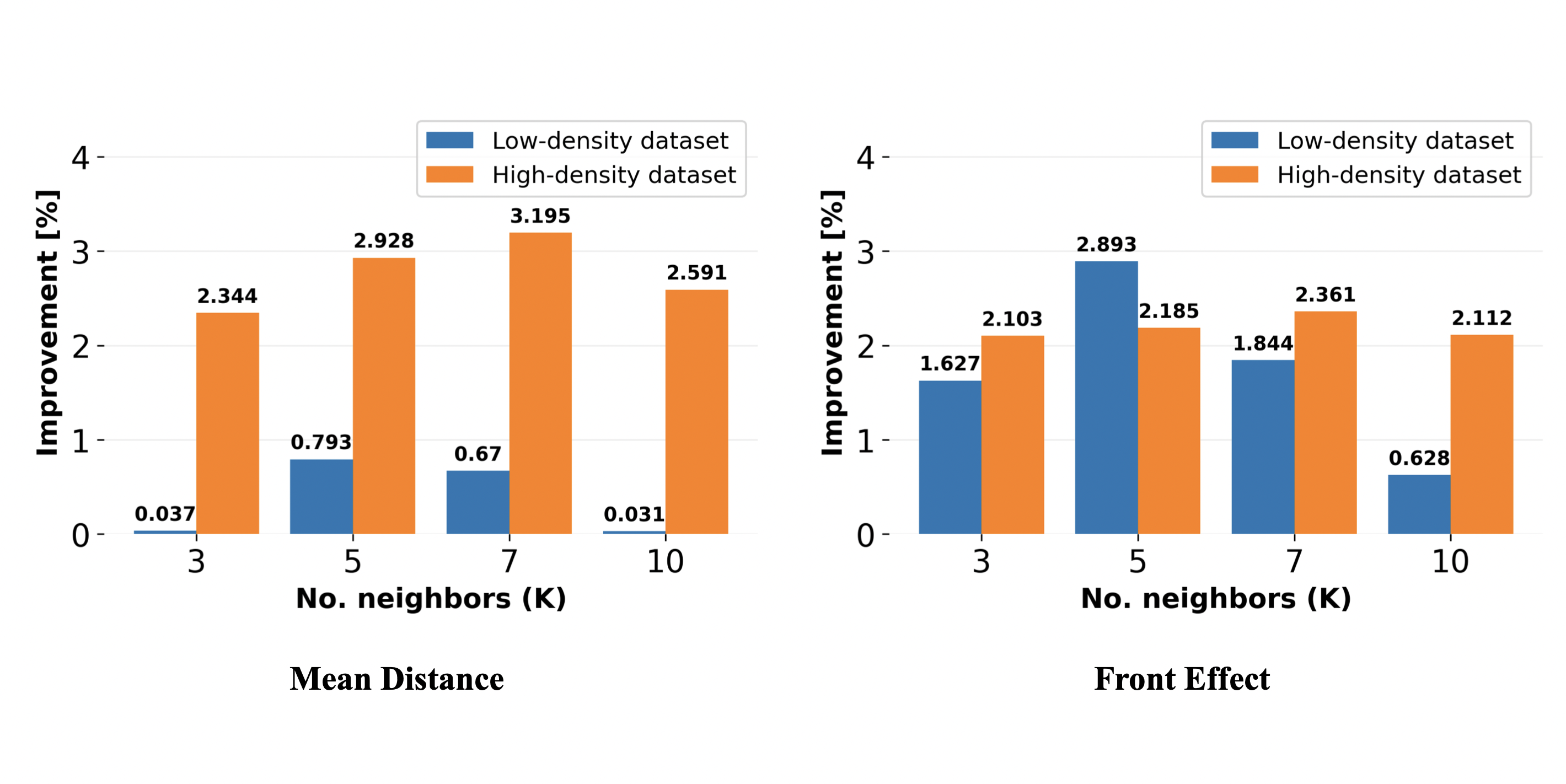
Downloads
Published
How to Cite
Issue
Section
License
Copyright (c) 2024 Huu-Tu Dang, Benoit Gaudou, Nicolas Verstaevel

This work is licensed under a Creative Commons Attribution 4.0 International License.
Authors contributing to Collective Dynamics agree to publish their articles under the Creative Commons Attribution 4.0 license.
This license allows:
Share — copy and redistribute the material in any medium or format
Adapt — remix, transform, and build upon the material
for any purpose, even commercially.
The licensor cannot revoke these freedoms as long as you follow the license terms.
Authors retain copyright of their work. They are permitted and encouraged to post items submitted to Collective Dynamics on personal or institutional websites and repositories, prior to and after publication (while providing the bibliographic details of that publication).